Ever wonder how we get reliable crowd answers in Stall Catchers? Well, there's a special kind of magic for that!
Our goal with Stall Catchers is to use crowd power to speed up the analysis of Alzheimer's disease research data. And we use crowdsourcing science to determine the best way to do that.
Everyone who's played Stall Catchers must have noticed, that movies usually have other answers from other catchers.
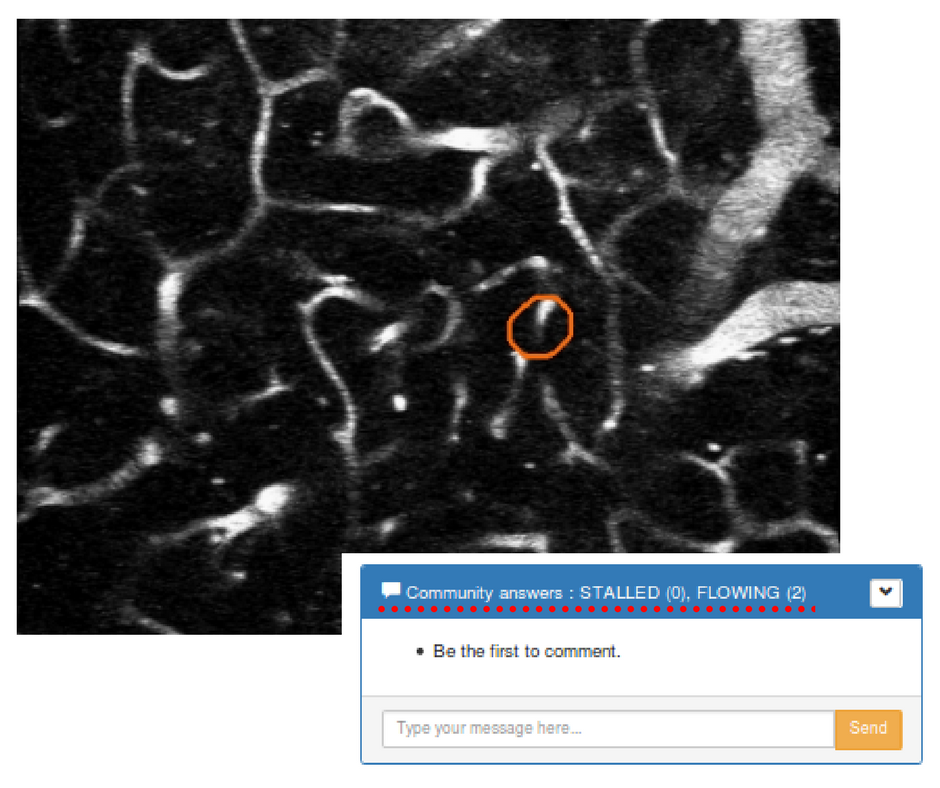
That's because, to get research quality data, we are combining answers about the same vessel from lots of participants. In other words, we don't assume that every catcher is as accurate as a trained lab technician. Instead we use "wisdom of crowd" methods that can derive one expert-like answer from many people.
The old example of this phenomenon comes from the weight-guessing game at the state fair – many people try to win the contest by guessing the weight of a person on display. They discovered that the average guess was always more reliable than any one person's guess.

So when we first validated Stall Catchers, our goal was to figure out how many answers about the same vessel had to be combined to consistently get a result as accurate as the lab technician's answer. And in this first attempt, we actually used a simple average – just like they did at the state fair! With this approach, we discovered that we needed to combine 20 answers to get a single "crowd answer" that is as good as the experts. So 20 was our "magic number".
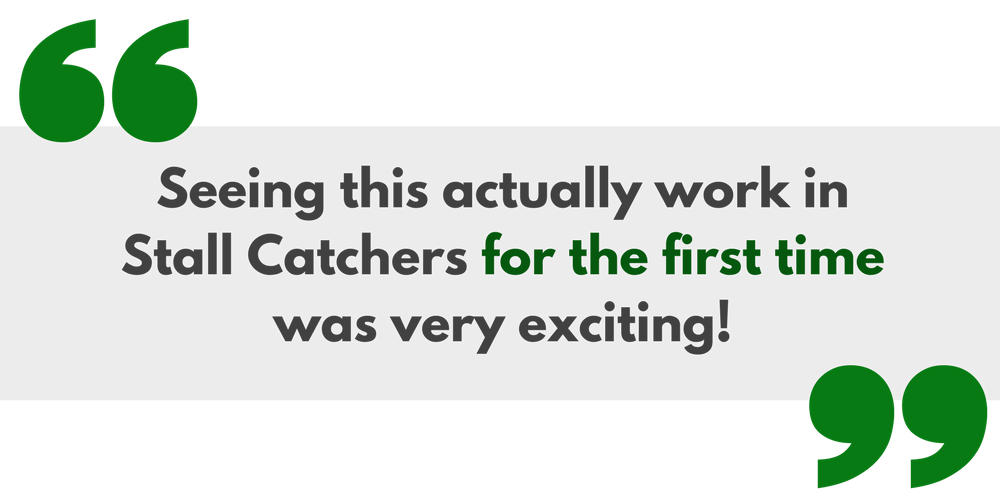
While this might not seem very efficient, it was a way to "prove the concept" – show that Stall Catchers, under the right conditions, could produce research quality data. Seeing this actually work for the first time was very exciting, and meant that we could then shift our focus to then making the system more efficient.
So our next step was to figure out how to reduce our magic number. For example, if we only had to combine 10 answers from Stall Catchers players to get one expert-like answer, it would double the value of each answer a person gave, and also cut in half the time to get to get the research done – in other words, to ultimately arrive at a treatment target.
We decided to take a two-prong approach. First, we realized that we might be able to get a crowd answer sooner by tracking answers as they arrived. If everyone is saying "stalled" about a vessel, maybe we don't need to wait for 20 answers that say "stalled" to realize that is a reliable crowd answer. So we came up with a way to measure a growing sense of confidence about a crowd answer, and then stop when that confidence gets high enough. Basically we said, "if our confidence in the crowd answer gets high enough, we don't need to collect any more answers for that vessel - we can move on to the next one". This is what we ultimately refer to as a dynamic stopping rule. And of course, we had to come up with a principled way of doing this and then validate the approach to make sure it worked.
Second, we realized that some people were more expert-like in their answers than others, either because they were more experienced in Stall Catchers or because it just came easily to them. Either way, we were already measuring this "sensitivity" for each user, which is represented by the "blue tube" that goes up and down over time depending on how well a catcher is answering training movies.
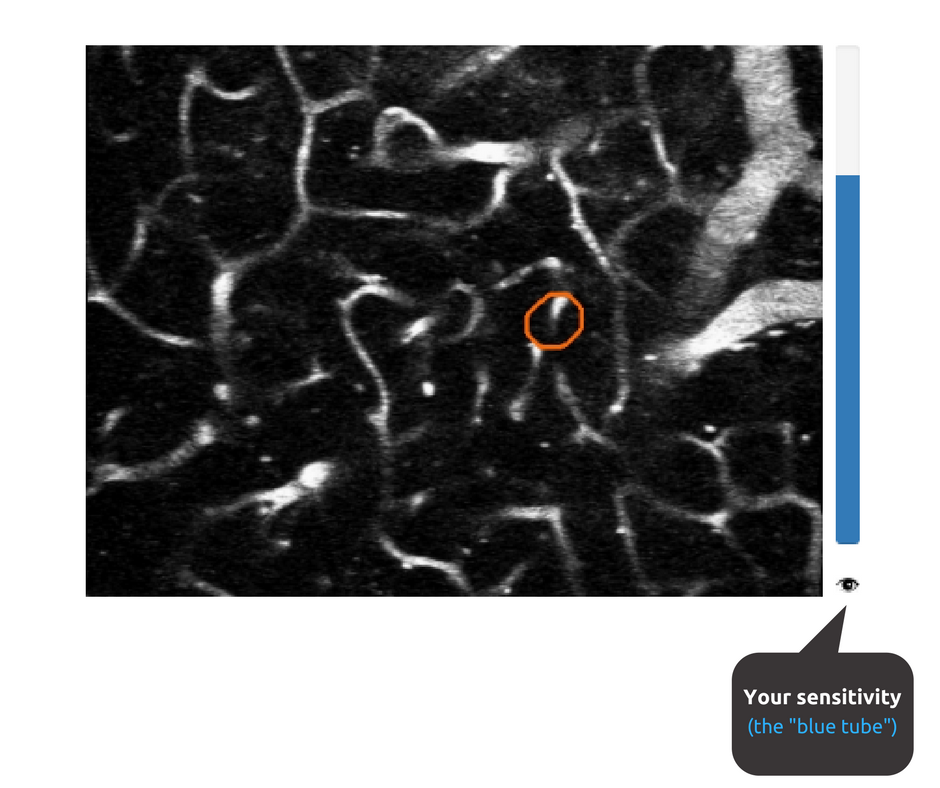
It occurred to us that if we assigned more weight to answers from experienced users than, say, new users, we might not need to combine as many answers to get an expert-like answer.
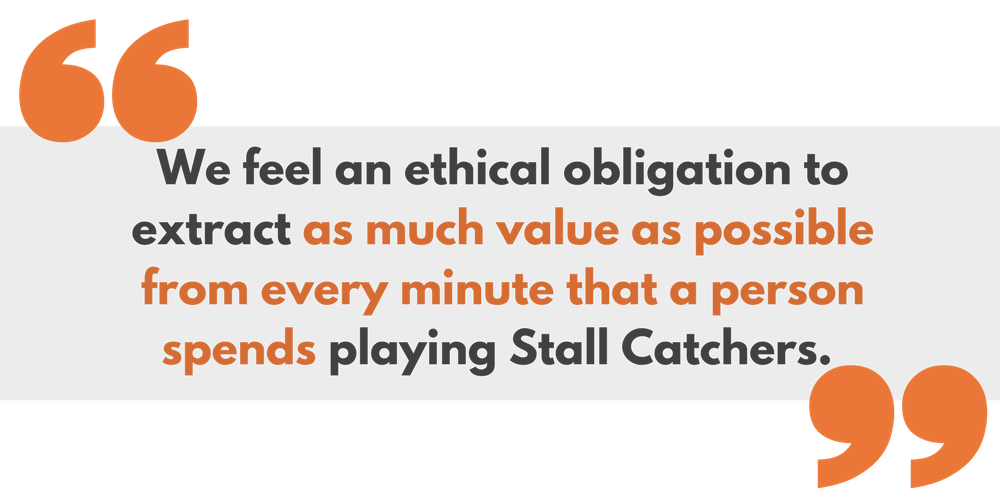
We combined these two methods and then conducted a new validation study to see what our new magic number might look like. Because we were using a dynamic stopping rule, there was no single magic number. Sometimes we might stop after only two very experienced users answered the same way, for others we might combine many answer from inexperienced users. What we found in the end was that our new method resulted in a range of 2 to 15 answers that needed to be combined, with a new (average) magic number of 7, which was almost three times more efficient than our first version!
We may be able to improve this further, but probably not by much, though we will continue trying, because we feel an ethical obligation to extract as much value as possible from every minute that a person spends playing Stall Catchers.
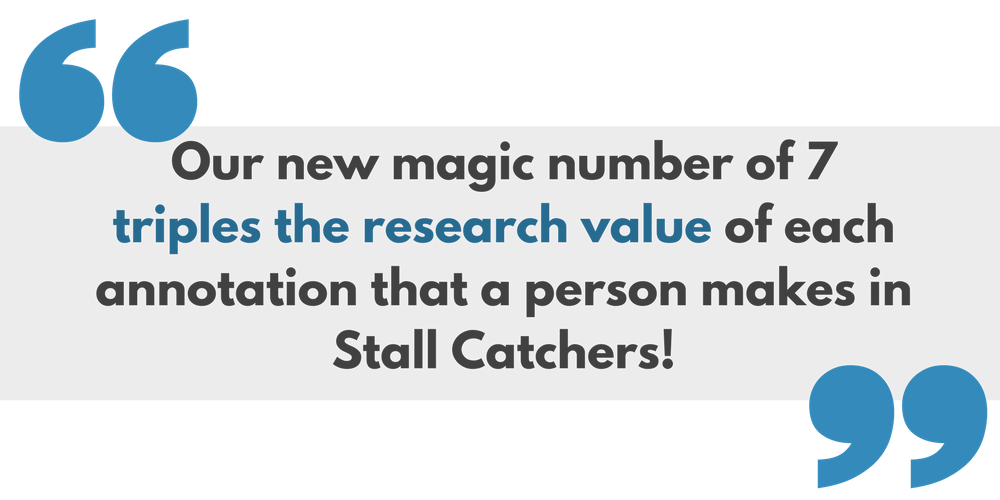
We are excited that this new methods triples the research value of each annotation that a person makes in Stall Catchers, and consequently reduces the overall research time to get to a potential Alzheimer's treatment by a factor of three!