You might be familiar with the Hippocratic Oath taken by physicians to āFirst do no harmā. My colleagues and I have adopted our own version of this: āFirst use no humansā, which means if there is a way to solve a problem using machines, we should not ask a human to do it.
This might seem counter intuitive given that our mission is ādedicated to the betterment of society through novel methods leveraging the complementary strengths of networked humans and machinesā. But we believe that sometimes action with the best of intentions can have costs that outweigh the benefits. Ā If there is a job that machines can do, we think it would be unethical to waste volunteer human cognitive labor on that job, when there are other, more pressing societal needs that require the unique mental faculties of the magnificent human mind.
Machine learning competition!

In 2017, the year after we launched Stall Catchers, I answered so many inquiries about using machine learning to do this task instead of burdening people, that I finally decided to write a blog post, explaining it once clearly for any future such inquiries. Ā
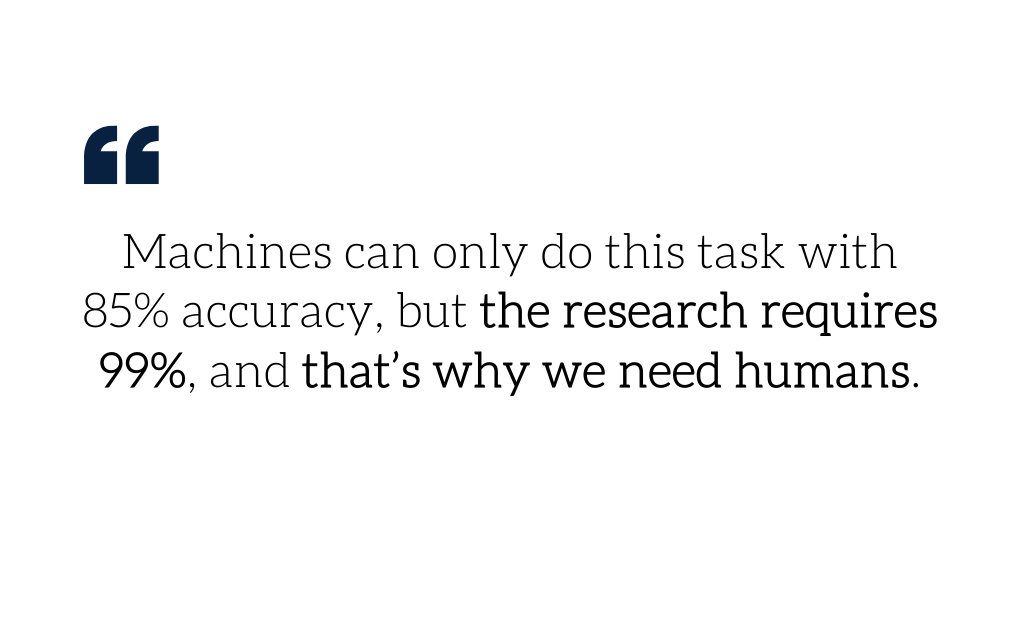
In that post, I explained that machines can only do this task with 85% accuracy, but the research requires 99% accuracy, and thatās why we need humans. Ā But that oversimplifies the situation.
Weāve always had a division of labor between machines and humans in Stall Catchers. Ā For example, the machines look through a huge digital image stack to find all the vessel segments and make movies with outlines drawn around them, which creates a single focused task that a human can easily respond to, labelling these vessel segments as āflowingā or āstalledā.
But why not give machines another chance?
Machine learning research is starved for huge labelled datasets. After almost 4 years of running Stall Catchers, we have over 500,000 vessel movies with millions of crowd-generated labels. This means that, for the first time ever, we can provide enough training data to give these systems a fresh chance to exceed the 85% accuracy levels they were able to achieve four years ago. Ā
Therefore we have a fantastic opportunity to invite a global community of highly engaged and creative machine learning researchers to create the best possible artificial intelligence system for analyzing our Alzheimerās research data. Ā
And... š„ Our new mission-aligned partner, Driven Data, has just launched a first-ever machine learning challenge using Stall Catchers data!
In the challenge, which will last until August 3, machine learning enthusiasts will compete for a $10,000 purse, generously donated by MathWorks - the company that created the famous data science programming language called MatLab.
You can learn more about the challenge - and join - here.
How will this work? Ā
We think we now have better conditions for finding a more accurate machine learning model that could be used on Stall Catchers data. But don't worry! Machines are unlikely to replace humans just yet.
The main goal of the competition is not just to find the model with the highest accuracy, but also one (or multiple ones) that can reliably tell us when it is 100% confident about its vessel movie labels.
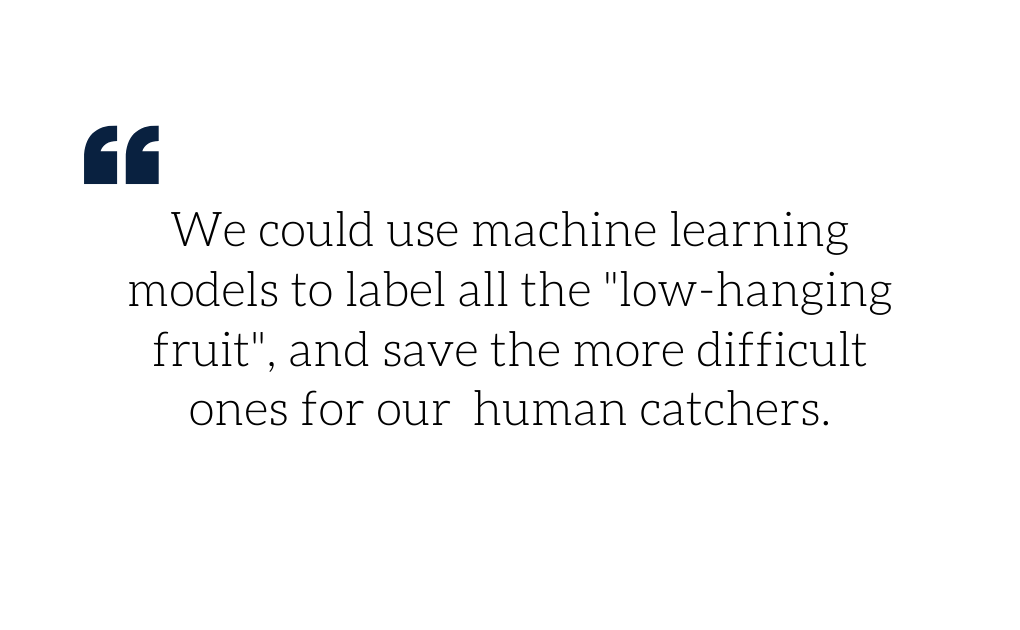
We could then use such models to label all the "low-hanging fruit" - the easier vessels, and save the more difficult ones for our Ā human catchers. In this arrangement, even if the new AI systems can only reliably label 20% of the images, thatās still 20% less time that volunteers would need to spend on a dataset, and a 20% speed up in the overall analysis time.
What comes next?
Depending on the machine learning models that come out, they could be integrated in various ways to the Stall Catchers analysis.
For instance, one outcome we are hoping for is "ensemble models".
In Stall Catchers, any given person rarely provides annotations consistently at the 99% accuracy level. But thatās OK, because we use āwisdom of crowdā methods to combine several answers into a single expert-like answer that does hit that high accuracy bar. (You can learn more about our wisdom of crowd methods here.)
Wisdom of crowds relies on the diversity of the crowd - everyone sees things a bit differently, and contributes in complementary ways. We could integrate different machine learning models in a similar way too. So even if none of the machine learning models are accurate enough on their own, we could create āensemble modelsā, which is basically using the wisdom of machines-based crowds.
Another configuration involves allowing each model developed in the competition to become a Stall Catchers user, with its own user account, playing alongside other catchers, and having its answers integrated with human answers using our existing wisdom of crowds methods. This could be groundbreaking for Human/AI research, and at the same time greatly speed up our analysis.
In the end, we may decide to even use a combination of these methods and others.
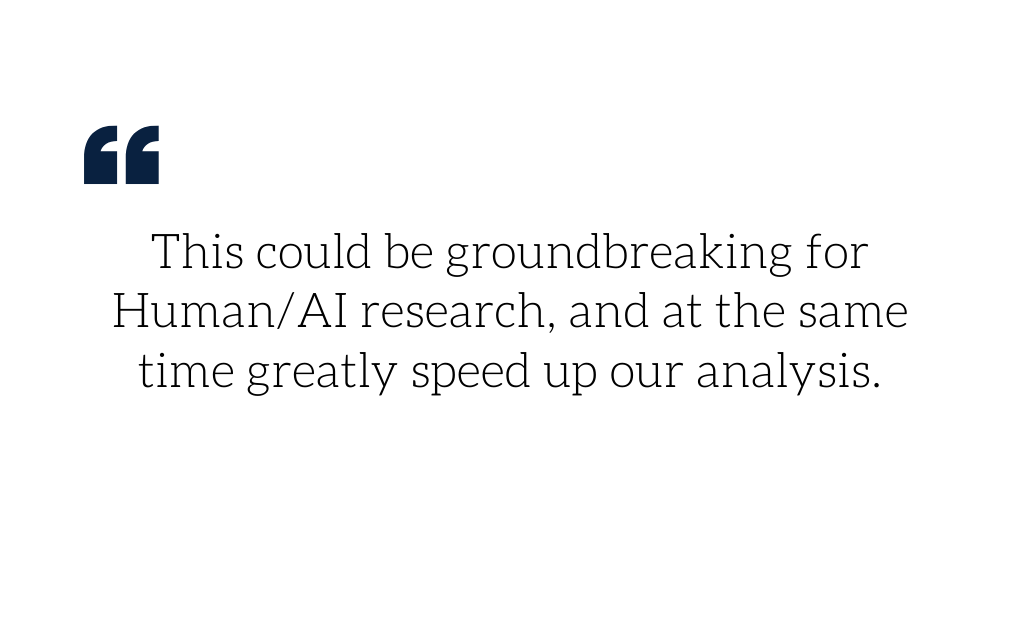
The bottom line is that when it comes to human computation - the science of designing systems of humans and machines that work together to solve problems, our goal is to push the envelope of how machines can work most effectively with humans. Ā
Such advancements will enable us to extract greater value from each human contribution and improve the overall performance of the system. Ideally, we seek to create a virtuous cycle in which the new data coming out of Stall Catchers will constantly feed and improve the artificial intelligence systems until eventually, machines will be able to do all the analysis, and humans can move onto our next challenge problems (hint: we have a close eye on Sickle Cell Disease).
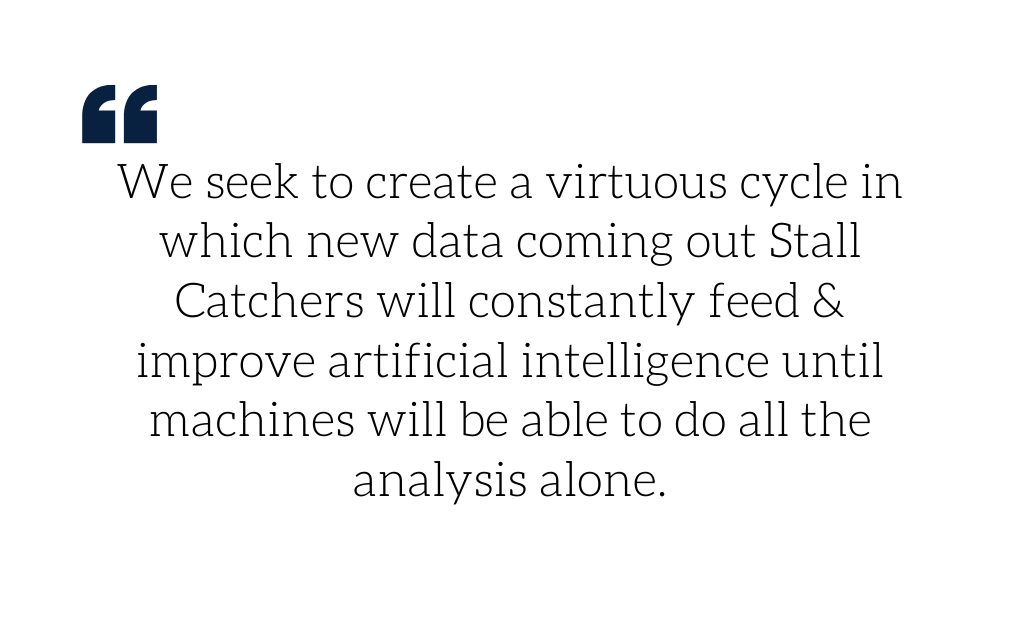
As you can imagine we will be monitoring closely the progress in this competition, and continue to report on progress and outcomes. Ā
This competition also happens to come at a time when the Schaffer-Nishimura laboratory at Cornell University, which uses Stall Catchers to speed up their analysis, is closer than ever to a mechanistic understanding of how capillary stalling occurs in mouse models of Alzheimerās disease.
So this could give us an exciting turbo-boost in rapidly answering the remaining research questions that will hopefully lead to a treatment for humans!
Find more info about the machine learning challenge, share and participate here.
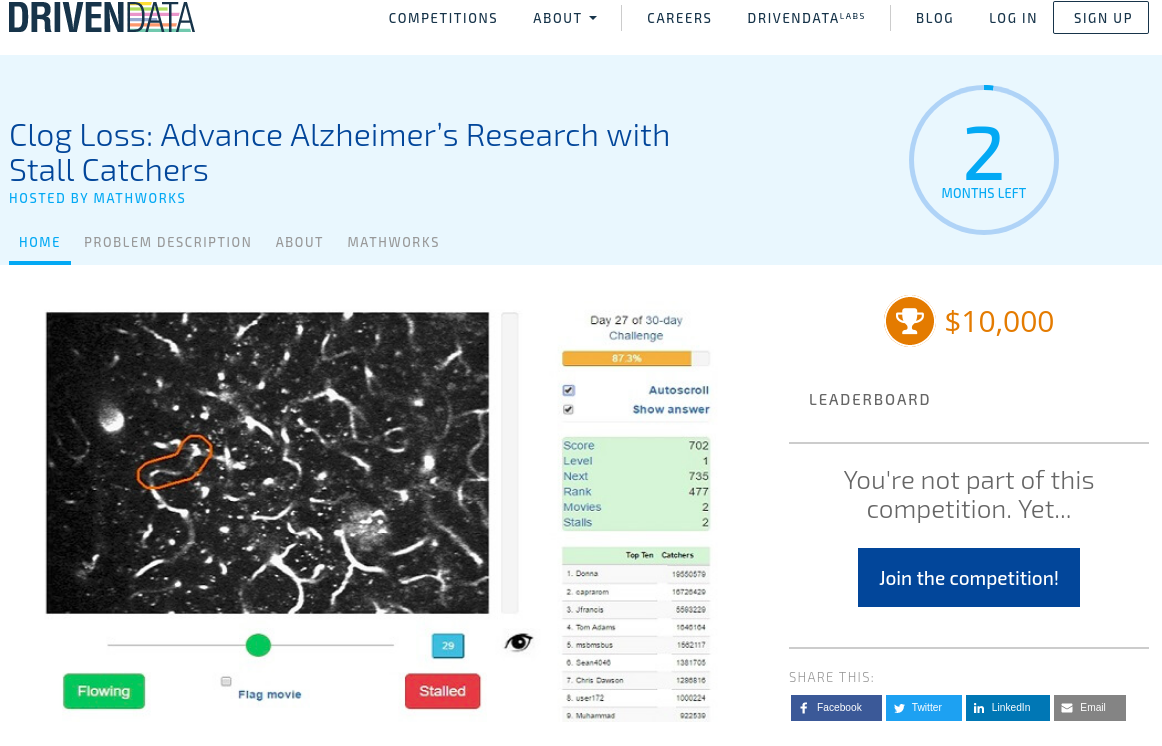
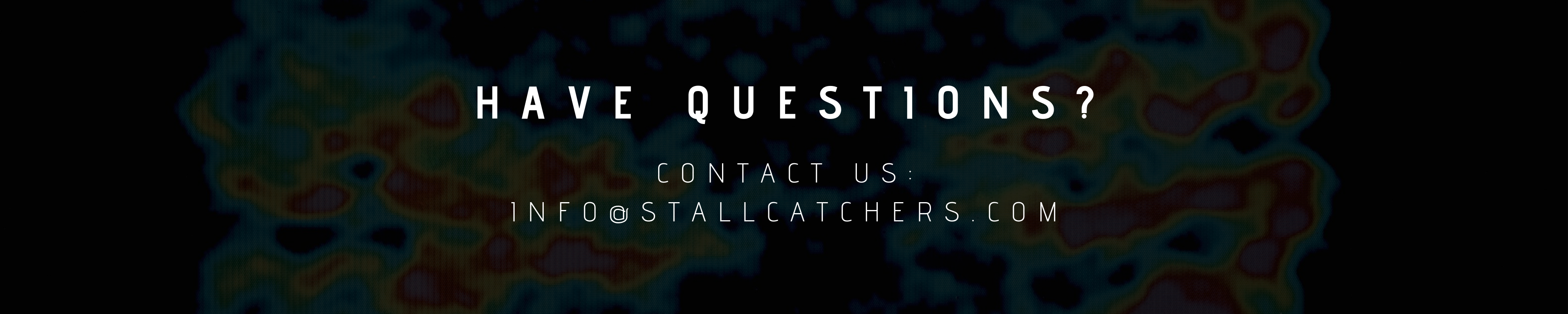