If we had a nickel for every time the question "why humans and not machines" has been asked in Stall Catchers history... :)
Well, the reasons why humans are so much better at catching stalls have been explained in this blog post very well. But it doesn't mean we stopped trying! If machines got better and could take some of the workload of us, that would speed up the research even more. And now, for the first time we have enough high quality catcher-generated data to train the machines and give them another chance!
To this end, our partners DrivenData have recently held a machine learning competition with Stall Catchers data.
In short: while there haven't been major artificial intelligence breakthroughs and machines are still falling quite a bit short of our stringent quality requirements in Stall Catchers, we got many excellent new algorithms entered into the competition, and we can use them in new and useful ways to speed up our search for an Alzheimer's treatment! Read on to find out how...
What was the competition for?
Anyone in the world who likes to experiment with machine learning and wants to try to build a model that can make predictions for a new kind of data (in this case, Alzheimer's research data with crowd answers as the ground truth).
For us it meant perhaps finding better models than those already tried (and failed) in automated Stall Catchers data analysis.
What were the results? Were the winning ML models better, than the ones already tried with Stall Catchers data?
We are almost certain that the winning models were better than the models previously tried with Stall Catchers, but since performance was measured differently we can't be 100% sure.
Either way, the winning models look like they are up to the job of speeding up Stall Catchers analysis because we now know how to combine these models in effective ways.
What are we going to do with the winning machine learning models?
We are going to use the winning models to annotate a bunch of the easier vessels so humans don't have to look at them. We need to validate this approach of course, but we think the winning models could analyze about 1/2 of Stall Catchers data - which would effectively double our analysis speed!
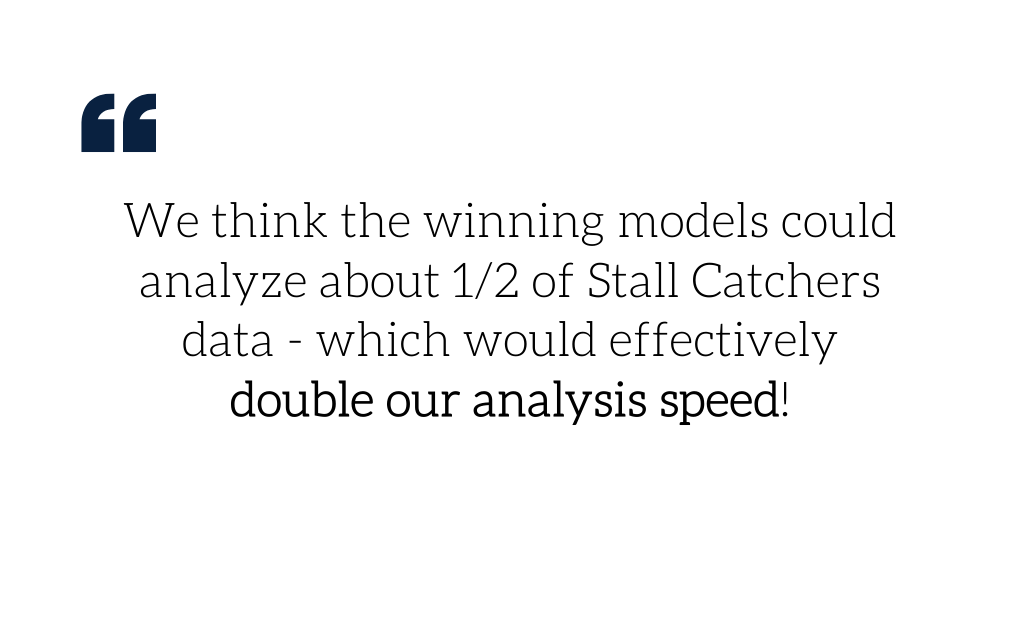
Then we will test out whether or not we can incorporate some of these models as actual stall catchers players for the rest of the vessels, and use their answers, just as we use individual catcher answers, to contribute to high quality crowd answers!
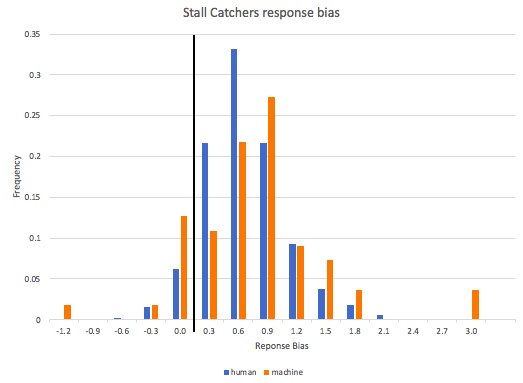
Although since they are machines and so fast - not to mention they don't sleep and could annotate around the clock - we might have to create a separate leaderboard for AI bots :)
What will the impact of that be to regular catchers?
If and when these new bot catchers join the game, regular catchers will see less boring (easy) vessels and get a higher percentage of stalls to look at - the more interesting ones! And the dataset progress bar will hopefully move twice as fast!
What will the impact be to the research?
Double the research speed, with no loss of quality!
How did catchers (catcher-generated data) support this machine learning competition and the resulting algorithms?
This competition would have been impossible without the millions of crowd answers provided by our catchers - all those data are needed to train the machine learning models. these machines learn by example, but they are SLOW learners and need LOTS of examples.
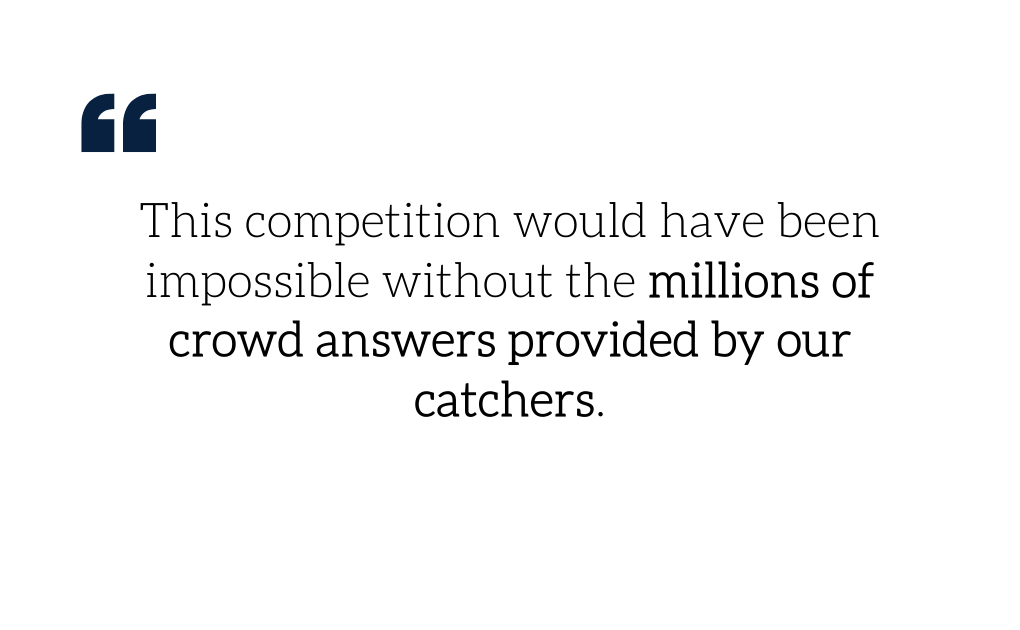
Will there be more such competitions? Will we try to continue to improve the machine learning models?
Yes, but we will have different competitions in the future that are more specifically targeted to certain capabilities. Eventually we hope to have machines that can do all of the Stall Catchers analysis, because we have new projects on the horizon with very different and quite interesting data where we will need to produce a similarly huge dataset to help teach machines. These new projects will address other disease research.
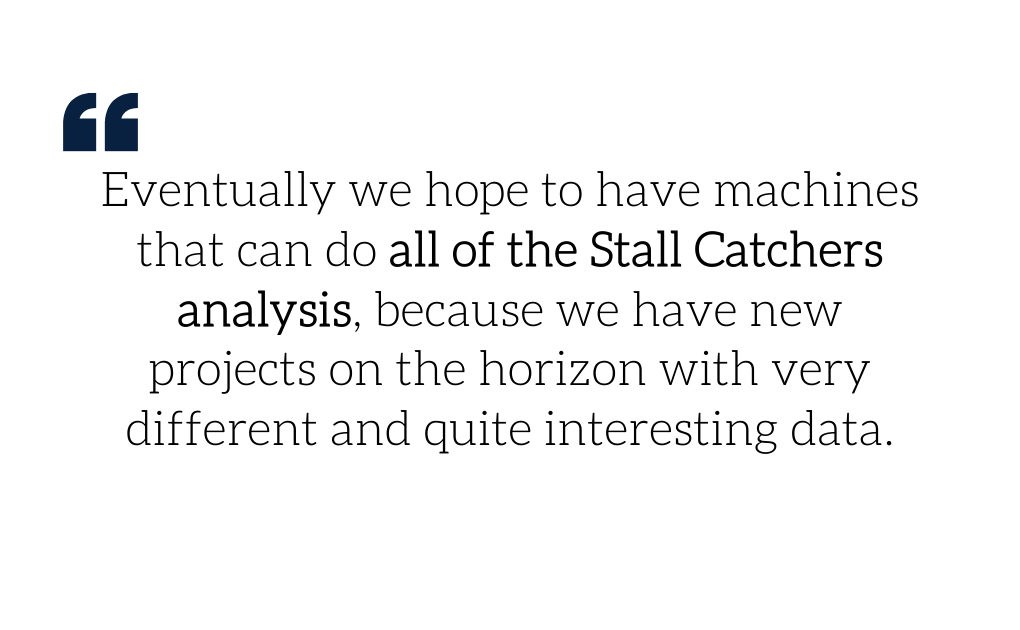
What was the general impression of the competition results and the winning models?
The winning models are way ahead of what we had before in terms of both performance and sophistication in the techniques they used. They resulted from tremendous creativity and perseverance.
You can learn more about the winners and prizes of the competition in this great blog post by DrivenData.